Overview
Using a base autonomous robotic team, researchers developed a paradigm that is easily scalable to multi-robot, multi-sensor autonomous teams. This paradigm can rapidly characterize an environment over a period of minutes and develop training data points for training other machine learning algorithms faster than ever before.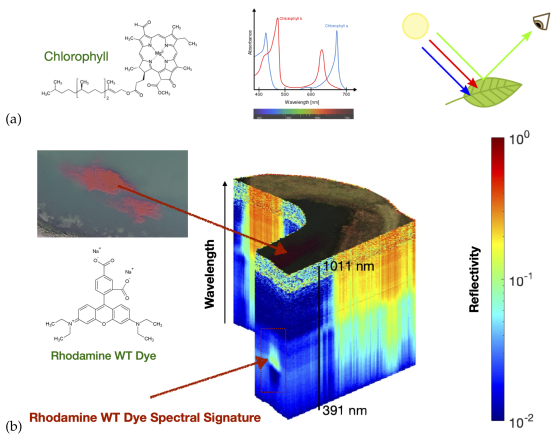
Figure 3. Panel (a) Chemicals absorb light in a characteristic way. Their absorption spectra is a function of their chemical structure. For every pixel we measure an entire spectrum with a hyper-spectral camera so we can identify chemicals within the scene. Panel (b) shows an example hyper-spectral data cube collected in North Texas on 23 November 2020. This particular data cube includes a simulant release, Rhodamine WT. The top layer of the hyper-spectral data cube shows the regular RGB image, the 462 stacked layers below show the reflectivity (on a log-scale) for each wavelength band between 391 and 1011 nm.